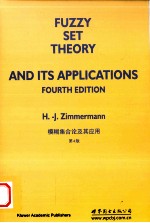
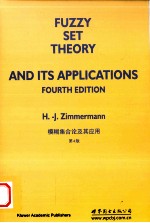
模糊集合论及其应用 第4版 英文PDF电子书下载
- 电子书积分:16 积分如何计算积分?
- 作 者:(美)齐默尔曼著
- 出 版 社:世界图书出版公司北京公司
- 出版年份:2011
- ISBN:7510035081
- 页数:514 页
1 Introduction to Fuzzy Sets 1
1.1 Crispness, Vagueness, Fuzziness, Uncertainty 1
1.2 Fuzzy Set Theory 2
PartⅠ: Fuzzy Mathematics 9
2 Fuzzy Sets-Basic Definitions 11
2.1 Basic Definitions 11
2.2 Basic Set-Theoretic Operations for Fuzzy Sets 16
3 Extensions 23
3.1 Types of Fuzzy Sets 23
3.2 Further Operations on Fuzzy Sets 27
3.2.1 Algebraic Operations 28
3.2.2 Set-Theoretic Operations 29
3.2.3 Criteria for Selecting Appropriate Aggregation Operators 43
4 Fuzzy Measures and Measures of Fuzziness 47
4.1 Fuzzy Measures 47
4.2 Measures of Fuzziness 49
5 The Extension Principle and Applications 55
5.1 The Extension Principle 55
5.2 Operations for Type 2 Fuzzy Sets 56
5.3 Algebraic Operations with Fuzzy Numbers 59
5.3.1 Special Extended Operations 61
5.3.2 Extended Operations for LR-Representation of Fuzzy Sets 64
6 Fuzzy Relations and Fuzzy Graphs 71
6.1 Fuzzy Relations on Sets and Fuzzy Sets 71
6.1.1 Compositions of Fuzzy Relations 76
6.1.2 Properties of the Min-Max Composition 79
6.2 Fuzzy Graphs 83
6.3 Special Fuzzy Relations 86
7 Fuzzy Analysis 93
7.1 Fuzzy Functions on Fuzzy Sets 93
7.2 Extrema of Fuzzy Functions 95
7.3 Integration of Fuzzy Functions 99
7.3.1 Integration of a Fuzzy Function over a Crisp Interval 100
7.3.2 Integration of a (Crisp) Real-Valued Function over a Fuzzy Interval 103
7.4 Fuzzy Differentiation 107
8 Uncertainty Modeling 111
8.1 Application-oriented Modeling of Uncertainty 111
8.1.1 Causes of Uncertainty 114
8.1.2 Type of Available Information 117
8.1.3 Uncertainty Methods 118
8.1.4 Uncertainty Theories as Transformers of Information 119
8.1.5 Matching Uncertainty Theory and Uncertain Phenomena 120
8.2 Possibility Theory 122
8.2.1 Fuzzy Sets and Possibility Distributions 122
8.2.2 Possibility and Necessity Measures 126
8.3 Probability of Fuzzy Events 129
8.3.1 Probability of a Fuzzy Event as a Scalar 129
8.3.2 Probability of a Fuzzy Event as a Fuzzy Set 131
8.4 Possibility vs.Probability 133
Part I: Applications of Fuzzy Set Theory 139
9 Fuzzy Logic and Approximate Reasoning 141
9.1 Linguistic Variables 141
9.2 Fuzzy Logic 149
9.2.1 Classical Logics Revisited 149
9.2.2 Linguistic Truth Tables 153
9.3 Approximate and Plausible Reasoning 156
9.4 Fuzzy Languages 160
9.5 Support Logic Programming and Fril 169
9.5.1 Introduction 169
9.5.2 Fril Rules 170
9.5.3 Inference Methods in Fril 172
9.5.4 Fril Inference for a Single Rule 175
9.5.5 Multiple Rule Case 176
9.5.6 Interval and Point Semantic Unification 177
9.5.7 Least Prejudiced Distribution and Learning 179
9.5.8 Applications of Fril 181
10 Fuzzy Sets and Expert Systems 185
10.1 Introduction to Expert Systems 185
10.2 Uncertainty Modeling in Expert Systems 193
10.3 Applications 203
11 Fuzzy Control 223
11.1 Origin and Objective 223
11.2 Automatic Control 225
11.3 The Fuzzy Controller 226
11.4 Types of Fuzzy Controllers 228
11.4.1 The Mamdani Controller 228
11.4.2 Defuzzification 232
11.4.3 The Sugeno Controller 239
11.5 Design Parameters 240
11.5.1 Scaling Factors 240
11.5.2 Fuzzy Sets 240
11.5.3 Rules 242
11.6 Adaptive Fuzzy Control 243
11.7 Applications 244
11.7.1 Crane Control 244
11.7.2 Control of a Model Car 246
11.7.3 Control of a Diesel Engine 248
11.7.4 Fuzzy Control of a Cement Kiln 249
11.8 Tools 255
11.9 Stability 257
11.10 Extensions 262
12 Fuzzy Data Bases and Queries 265
12.1 Introduction 265
12.2 Fuzzy Relational Databases 266
12.3 Fuzzy Queries in Crisp Databases 268
13 Fuzzy Data Analysis 277
13.1 Introduction 277
13.2 Methods for Fuzzy Data Analysis 279
13.2.1 Algorithmic Approaches 281
13.2.2 Knowledge-Based Approaches 302
13.2.3 Neural Net Approaches 304
13.3 Dynamic Fuzzy Data Analysis 306
13.3.1 Problem Description 306
13.3.2 Similarity of Functions 307
13.3.3 Approaches for Analysic Dynamic Systems 313
13.4 Tools for Fuzzy Data Analysis 317
13.4.1 Requirements for FDA Tools 317
13.4.2 Data Engine 318
13.5 Applications of FDA 322
13.5.1 Maintenance Management in Petrochemical Plants 322
13.5.2 Acoustic Quality Control 323
14 Decision Making in Fuzzy Environments 329
14.1 Fuzzy Decisions 329
14.2 Fuzzy Linear Programming 336
14.2.1 Symmetric Fuzzy LP 337
14.2.2 Fuzzy LP with Crisp Objective Function 342
14.3 Fuzzy Dynamic Programming 348
14.3.1 Fuzzy Dynamic Programming with Crisp State Transformation Function 349
14.4 Fuzzy Multicriteria Analysis 352
14.4.1 Multi Objective Decision Making (MODM) 353
14.4.2 Multi Attributive Decision Making (MADM) 359
15 Applications of Fuzzy Sets in Engineering and Management 371
15.1 Introduction 371
15.2 Engineering Applications 373
15.2.1 Linguistic Evaluation and Ranking of Machine Tools 375
15.2.2 Fault Detection in Gearboxes 381
15.3 Applications in Management 389
15.3.1 A Discrete Location Model 390
15.3.2 Fuzzy Set Models in Logistics 393
15.3.2.1 Fuzzy Approach to the Transportation Problem 393
15.3.2.2 Fuzzy Linear Programming in Logistics 398
15.3.3 Fuzzy Sets in Scheduling 401
15.3.3.1 Job-Shop Scheduling with Expert Systems 401
15.3.3.2 A Method to Control Flexible Manufacturing Systems 405
15.3.3.3 Aggregate Production and Inventory Planning 411
15.3.3.4 Fuzzy Mathematical Programming for Maintenance Scheduling 418
15.3.3.5 Scheduling Courses, Instructors, and Classrooms 419
15.3.4 Fuzzy Set Models in Inventory Control 426
15.3.5 Fuzzy Sets in Marketing 432
15.3.5.1 Customer Segmentation in Banking and Finance 432
15.3.5.2 Bank Customer Segmentation based on Customer Behavior 433
16 Empirical Research in Fuzzy Set Theory 443
16.1 Formal Theories vs& Factual Theories vs.Decision Technologies 443
16.1.1 Models in Operations Research and Management Science 447
16.1.2 Testing Factual Models 449
16.2 Empirical Research on Membership Functions 453
16.2.1 Type A-Membership Model 454
16.2.2 Type B-Membership Model 456
16.3 Empirical Research on Aggregators 463
16.4 Conclusions 474
17 Future Perspectives 477
Abbreviations of Frequently Cited Journals 481
Bibliography 483
Index 507
Figure 1-1 Concept hierarchy of creditworthiness. 5
Figure 2-1 Real numbers close to 10. 13
Figure 2-2a Convex fuzzy set. 15
Figure 2-2b Nonconvex fuzzy set. 15
Figure 2-3 Union and intersection of fuzzy sets. 18
Figure 3-1 Fuzzy sets vs.probabilistic sets. 26
Figure 3-2 Mapping of t-norms,t-conorms, and averaging operators. 38
Figure 5-1 The extension principle. 57
Figure 5-2 Trapezoidal “fuzzy number”. 60
Figure 5-3 LR representation of fuzzy numbers. 65
Figure 6-1 Fuzzy graphs. 84
Figure 6-2 Fuzzy forests. 86
Figure 6-3 Graphs that are not forests. 86
Figure 7-1 Maximizing set. 96
Figure 7-2 A fuzzy function. 97
Figure 7-3 Triangular fuzzy numbers representing a fuzzy function. 98
Figure 7-4 The maximum of a fuzzy function. 99
Figure 7-5 Fuzzily bounded interval. 104
Figure 8-1 Uncertainty as situational property. 113
Figure 8-2 Probability of a fuzzy event. 134
Figure 9-1 Linguistic variable “Age”. 143
Figure 9-2 Linguistic variable “Probability. 144
Figure 9-3 Linguistic variable “Truth”. 145
Figure 9-4 Terms “True” and “False”. 146
Figure 10-1 Structure of an expert system. 189
Figure 10-2 Semantic net. 191
Figure 10-3 Linguistic descriptors. 205
Figure 10-4 Label sets for semantic representation. 205
Figure 10-5 Linguistic variables for occurrence and confirmability. 209
Figure 10-6 Inference network for damage assessment of existing structures [Ishizuka et al.1982, p.263]. 212
Figure 10-7 Combination of two two-dimensional portfolios. 215
Figure 10-8 Criteria tree for technology attractiveness. 216
Figure 10-9 Terms of “degree of achievement”. 217
Figure 10-10 Aggregation of linguistic variables. 218
Figure 10-11 Portfolio with linguistic input. 220
Figure 10-12 Structure of ESP. 221
Figure 11-1 Automatic feedback control. 225
Figure 11-2 Generic Mamdani fuzzy controller. 227
Figure 11-3 Linguistic variable “Temperature”. 229
Figure 114Rule consequences in the heating system example. 232
Figure 115Extreme Value Strategies. 234
Figure 116COA Defuzzification. 235
Figure 11-7 Neighboring membership functions. 236
Figure 118Separate membership functions. 236
Figure 119Parameters describing fuzzy sets. 241
Figure 11-10 Influence of symmetry. 242
Figure 11-11 Condition width. 242
Figure 11-12 Container crane [von Altrock 1993]. 245
Figure 11-13 Phases of motion. 245
Figure 11-14 Input variables [Sugeno and Nishida 1985, p.106]. 246
Figure 11-15 Trajectories of the fuzzy controlled model car [Sugeno and Nishida 1985, p.112]. 247
Figure 11-16 Fuzzy model car [von Altrock et al.1992, p.42]. 248
Figure 11-17 Experimental design [von Altrock et al.1992, p.48]. 249
Figure 11-18 FCR vs.fuel injection timing [Murayama et al.1985, p.64]. 250
Figure 11-19 Control algorithm [Murayama et al.1985]. 251
Figure 11-20 Experimental results [Murayama et al.1985]. 252
Figure 11-21 Schematic diagram of rotary cement kiln [Umbers andKing 1981,p.371]. 252
Figure 11-22 Controller development in fuzzyTECH [von Altrock et al.1992]. 256
Figure 11-23 Rule base for model car [von Altrock et al.1992]. 256
Figure 11-24 Simulation screen [von Altrock et al.1992]. 257
Figure 11-25 Fuzzy controller as a nonlinear transfer element. 258
Figure 11-26 Classification of stability analysis approaches. 259
Figure 1127 Linguistic state space. 260
Figure 11-28 Linguistic trajectory. 261
Figure 13-1 Scope of data analysis. 280
Figure 13-2 Possible data structure in the plane. 282
Figure 13-3 Performance of cluster criteria. 283
Figure 13-4 Dendogram for hierarchical clusters. 283
Figure 13-5 Fuzzy graph. 285
Figure 13-6 Dendogram for graph-theoretic clusters. 285
Figure 13-7 The butterfly. 286
Figure 13-8 Crisp clusters of the butterfly. 287
Figure 13-9 Cluster 1 of the butterfly. 287
Figure 13-10 Cluster 2 of the butterfly. 288
Figure 13-11 Clusters for m=1.25. 295
Figure 13-12 Clusters for m=2. 295
Figure 13-13 Clusters by the FSC.(a) Data set; (b) circles found by FSC;(c)data set;(d)circles found by FSC. 300
Figure 13-14 Data sets [Krishnapuram and Keller 1993]. 301
Figure 13-15 Knowledge-based classification. 303
Figure 13-16 Linguistic variables “Depth of Cut” and “Feed”. 304
Figure 13-17 Knowledge base. 304
Figure 13-18 Basic structure of the knowledge-based system. 305
Figure 13-19 (a) States of objects at a point of time;(b) projections of trajectories over time into the feature space. 307
Figure 13-20 Structural and pointwise similarity. 308
Figure 13-21 Fictitious developments of share prices. 309
Figure 13-22 Idealized characteristic patterns of time signals for (a)an intact engine; (b) an engine with some defect. 309
Figure 13-23 (a) The fuzzy set “approximately zero” (μ(y)), the function f(t) and the resulting pointwise similarityμ(f(t));(b)projection of pointwise similarity into the plane (t,μ(f(t))). 311
Figure 13-24 Transformation of a feature vector containing trajectories into trajectories into a usual feature vector. 314
Figure 13-25 Input and output of the functional fuzzy c-means. 315
Figure 13-26 Structure of DataEngine. 318
Figure 13-27 Screen shot of DataEngine. 320
Figure 13-28 Cracking furnace. 324
Figure 13-29 Furnace temperature. 325
Figure 13-30 Fuzzy classification of continuous process. 325
Figure 13-31 Application of DataEngine for acoustic quality control. 327
Figure 14-1 A classical decision under certainty. 330
Figure 14-2 A fuzzy decision. 332
Figure 14-3 Optimal dividend as maximizing decision. 333
Figure 14-4 Feasible regions for μ?(x)=0 and μ?(x)=1 344
Figure 14-5 Fuzzy decision. 345
Figure 14-6 Basic structure of a dynamic programming model. 349
Figure 14-7 The vector-maximum problem. 355
Figure 14-8 Fuzzy LP with min-operator. 357
Figure 14-9 Fuzzy sets representing weights and ratings. 366
Figure 14-10 Final ratings of alternatives. 368
Figure 14-11 Preferability of alternative 2 over all others. 369
Figure 15-1 Linguistic values for variable “rigidity. 376
Figure 15-2 Linguistic values for variable “elements' rigidity”. 377
Figure 15-3 Linguistic values for variable “significance”. 379
Figure 15-4 Linguistic evaluation values of lathes B,C,D,E. 380
Figure 15-5 Membership functions resulting from incremental classifier design and classification of data obtained till point 440. 384
Figure 15-6 Membership functions for time window 〈230,330〉. 385
Figure 15-7 Membership functions for time window 〈240,340〉. 386
Figure 15-8 Membership functions for time window 〈250,350〉. 386
Figure 15-9 Proportional difference between class centers 1 and 2(with respect to the center of class 2) in time window〈250,350〉. 387
Figure 15-10 Membership functions for time window 〈3014,3114〉. 388
Figure 15-11 Membership functions for time window 〈3064,3200〉. 388
Figure 15-12 Road network. 392
Figure 15-13 Feasible covers. 392
Figure 15-14 i ne trapezoidai form of a fuzzy number ai=(ai1,ai1,ai2,ai-2). 394
Figure 15-15 The membership function of the fuzzy goal G. 394
Figure 15-16 The solution of the numerical example. 399
Figure 15-17 Structure of OPAL. 402
Figure 15-18 Fuzzy sets for the ratio in the “if”part of the rules. 404
Figure 15-19 Example of an FMS [Hartley 1984, p.194]. 405
Figure 15-20 Criteria hierarchies.(a) Release scheduling; (b)Machine scheduling. 407
Figure 15-21 Principle of approximate reasoning. 409
Figure 15-22 Membership functions for several linguistic terms. 413
Figure 15-23 Comparison of work force algorithms. 416
Figure 15-24 Flowtime of a course. 421
Figure 15-25 The scheduling process. 422
Figure 15-26 Courses of one instruction program. 424
Figure 15-27 Feature 1:current end-of-month balance for“Y”. 438
Figure 15-28 Feature 1: current end-of-month balance for“N”. 439
Figure 16-1 Calibration of the interval for measurement. 458
Figure 16-2 Subject 34, “Old Man”. 460
Figure 16-3 Subject 58, “Very Old Man”. 461
Figure 16-4 Subject 5, “Very Young Man”. 461
Figure 16-5 Subject 15, “Very Young Man”. 462
Figure 16-6 Subject 17, “Young Man”. 462
Figure 16-7 Subject 32, “Young Man”. 463
Figure 16-8 Empirical membership functions “Very Young Man”,“Young Man”,“Old Man”,“Very Old Man”. 464
Figure 16-9 Empirical unimodel membership functions“Very Young Man”,“Young Man”. 464
Figure 16-10 Min-operator: Observed vs.expected grades of membership. 468
Figure 16-11 Product-operator: Observed vs.expected grades of membership. 469
Figure 16-12 Predicted vs.observed data: Min-operator. 472
Figure 16-13 Predicted vs.observed data: Max-operator. 473
Figure 16-14 Predicted vs.observed data: Geometric mean operator. 473
Figure 16-15 Predicted vs.observed data: γ-operator. 474
Figure 16-16 Concept hierarchy of creditworthiness together with individual weights d and g-values for each level of aggregation. 475
Table 3-1 Classification of compensatory and noncompensatory operators. 39
Table 3-2 Classification of aggregation operators. 40
Table 3-3 Relationship between parameterized operators and their parameters. 41
Table 6-1 Properties of fuzzy relations. 89
Table 8-1 Rough taxonomy of uncertainty properties. 121
Table 8-2 Possibility functions. 128
Table 8-3 Koopman's vs.Kolmogoroff's probabilities. 136
Table 8-4 Relationship between Boolean algebra, probabilities,and possibilities. 137
Table 9-1 Formal quality of implication operators. 158
Table 10-1 Expert systems. 192
Table 10-2 A crisp data base. 196
Table 10-3 An extended data base. 196
Table 10-4 A possibilistic data base. 199
Table 10-5 α-level sets. 201
Table 11-1 Rule base. 230
Table 11-2 Properties of defuzzifiers. 238
Table 14-1 Ratings and weights of alternative goals. 367
Table 15-1 Selected applications in management and engineering. 374
Table 15-2 Experimental Data. 376
Table 15-3 Surface quality parameters (output data). 376
Table 15-4 Boundary values of the linguistic variable“significance”. 378
Table 15-5a Populations. 391
Table 15-5b Distances between villages. 391
Table 15-6 Determination of the fuzzy set decision. 393
Table 15-7 Table of the parametric transportation problem. 397
Table 15-8 Solution to transportation problem. 398
Table 15-9 Membership grades for slack time and waiting time. 410
Table 15-10 Membership grades for conditional parts of the rules. 411
Table 15-11 Membership grades for the rules. 411
Table 15-12 Results. 412
Table 15-13 Definition of linguistic variables [Rinks 1982]. 414
Table 15-14 Membership functions. 415
Table 15-15 Cost results. 417
Table 15-16 Comparison of performances. 417
Table 15-17 Structure of instruction program. 423
Table 15-18 Availability of instructors. 425
Table 15-19 PERT output. 425
Table 15-20 Availability of weeks for courses. 426
Table 15-21 First week's final schedule. 426
Table 15-22 Cluster centers of nine optimal classes. 434
Table 15-23 Dynamic features describing bank customers. 434
Table 15-24 Main statistics of each feature of the data group“Y”. 435
Table 15-25 Main statistics of each feature of data group “N”. 435
Table 15-26 Scope of the analysis of bank customers. 436
Table 15-27 Absorbed and stray customers for “Y”-group. 437
Table 15-28 Absorbed and stray customers for “N”-group. 438
Table 15-29 Temporal change of assignment of customers in group“Y”to clusters. 439
Table 15-30 Temporal change of assignment of customers in group“N”to clusters. 439
Table 16-1 Hierarchy of scale levels. 451
Table 16-2 Empirically determined grades of membership. 455
Table 16-3 Empirical vs.predicted grades of membership. 467
- 《钒产业技术及应用》高峰,彭清静,华骏主编 2019
- 《现代水泥技术发展与应用论文集》天津水泥工业设计研究院有限公司编 2019
- 《英汉翻译理论的多维阐释及应用剖析》常瑞娟著 2019
- 《数据库技术与应用 Access 2010 微课版 第2版》刘卫国主编 2020
- 《区块链DAPP开发入门、代码实现、场景应用》李万胜著 2019
- 《虚拟流域环境理论技术研究与应用》冶运涛蒋云钟梁犁丽曹引等编著 2019
- 《当代翻译美学的理论诠释与应用解读》宁建庚著 2019
- 《第一性原理方法及应用》李青坤著 2019
- 《教师教育系列教材 心理学原理与应用 第2版 视频版》郑红,倪嘉波,刘亨荣编;陈冬梅责编 2020
- 《物联网与嵌入式技术及其在农业上的应用》马德新 2019
- 《TED说话的力量 世界优秀演讲者的口才秘诀》(坦桑)阿卡什·P.卡里亚著 2019
- 《小手画出大世界 恐龙世界》登亚编绘 2008
- 《近代世界史文献丛编 19》王强主编 2017
- 《课堂上听不到的历史传奇 世界政治军事名人 初中版》顾跃忠等编著 2015
- 《指向核心素养 北京十一学校名师教学设计 英语 七年级 上 配人教版》周志英总主编 2019
- 《365奇趣英语乐园 世界民间故事》爱思得图书国际企业 2018
- 《近代世界史文献丛编 36》王强主编 2017
- 《北京生态环境保护》《北京环境保护丛书》编委会编著 2018
- 《近代世界史文献丛编 11》王强主编 2017
- 《近代世界史文献丛编 18》王强主编 2017