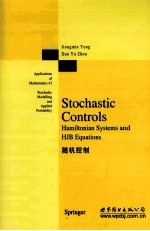
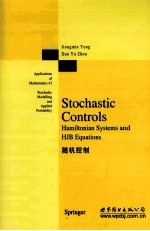
随机控制 英文PDF电子书下载
- 电子书积分:14 积分如何计算积分?
- 作 者:雍炯敏著
- 出 版 社:北京/西安:世界图书出版公司
- 出版年份:2012
- ISBN:9787510048029
- 页数:440 页
Chapter 1.Basic Stochastic Calculus 1
1.Probability 1
1.1.Probability spaces 1
1.2.Random variables 4
1.3.Conditional expectation 8
1.4.Convergence of probabilities 13
2.Stochastic Processes 15
2.1.General considerations 15
2.2.Brownian motions 21
3.Stopping Times 23
4.Martingales 27
5.It?'s Integral 30
5.1.Nondifferentiability of Brownian motion 30
5.2.Definition of It?'s integral and basic properties 32
5.3.It?'s formula 36
5.4.Martingale representation theorems 38
6.Stochastic Differential Equations 40
6.1.Strong solutions 41
6.2.Weak solutions 44
6.3.Linear SDEs 47
6.4.Other types of SDEs 48
Chapter 2.Stochastic Optimal Control Problems 51
1.Introduction 51
2.Deterministic Cases Revisited 52
3.Examples of Stochastic Control Problems 55
3.1.Production planning 55
3.2.Investment vs.consumption 56
3.3.Reinsurance and dividend management 58
3.4.Technology diffusion 59
3.5.Queueing systems in heavy traffic 60
4.Formulations of Stochastic Optimal Control Problems 62
4.1.Strong formulation 62
4.2.Weak formulation 64
5.Existence of Optimal Controls 65
5.1.A deterministic result 65
5.2.Existence under strong formulation 67
5.3.Existence under weak formulation 69
6.Reachable Sets of Stochastic Control Systems 75
6.1.Nonconvexity of the reachable sets 76
6.2.Noncloseness of the reachable sets 81
7.Other Stochastic Control Models 85
7.1.Random duration 85
7.2.Optimal stopping 86
7.3.Singular and impulse controls 86
7.4.Risk-sensitive controls 88
7.5.Ergodic controls 89
7.6.Partially observable systems 89
8.Historical Remarks 92
Chapter 3.Maximum Principle and Stochastic Hamiltonian Systems 101
1.Introduction 101
2.The Deterministic Case Revisited 102
3.Statement of the Stochastic Maximum Principle 113
3.1.Adjoint equations 115
3.2.The maximum principle and stochastic Hamiltonian systems 117
3.3.A worked-out example 120
4.A Proof of the Maximum Principle 123
4.1.A moment estimate 124
4.2.Taylor expansions 126
4.3.Duality analysis and completion of the proof 134
5.Sufficient Conditions of Optimality 137
6.Problems with Statc Constraints 141
6.1.Formulation of the problem and the maximum principle 141
6.2.Some preliminary lemmas 145
6.3.A proof of Theorem 6.1 149
7.Historical Remarks 153
Chapter 4.Dynamic Programming and HJB Equations 157
1.Introduction 157
2.The Detcrministic Case Revisited 158
3.The Stochastic Principle of Optimality and the HJB Equation 175
3.1.A stochastic framework for dynamic programming 175
3.2.Principle of optimality 180
3.3.The HJB equation 182
4.Other Properties of the Value Function 184
4.1.Continuous dependence on parameters 184
4.2.Semiconcavity 186
5.Viscosity Solutions 189
5.1.Definitions 189
5.2.Some properties 196
6.Uniqueness of Viscosity Solutions 198
6.1.A uniqueness theorem 198
6.2.Proofs of Lemmas 6.6 and 6.7 208
7.Historical Remarks 212
Chapter 5.The Relationship Between the Maximum Principle and Dynamic Programming 217
1.Introduction 217
2.Classical Hamilton-Jacobi Thcory 219
3.Relationship for Deterministic Systems 227
3.1.Adjoint variable and value function:Smooth case 229
3.2.Economic interpretation 231
3.3.Methods of characteristics and the Feynman-Kac formula 232
3.4.Adjoint variable and value function:Nonsmooth case 235
3.5.Vcrification theorems 241
4.Relationship for Stochastic Systems 247
4.1.Smooth case 250
4.2.Nonsmooth case:Differentials in the spatial variable 255
4.3.Nonsmooth case:Differentials in the time variable 263
5.Stochastic Vcrification Theorems 268
5.1.Smooth case 268
5.2.Nonsmooth case 269
6.Optimal Feedback Controls 275
7.Historical Remarks 278
Chapter 6.Linear Quadratic Optimal Control Problems 281
1.Introduction 281
2.The Deterministic LQ Problems Revisited 284
2.1.Formulation 284
2.2.A minimization problem of a quadratic functional 286
2.3.A linear Hamiltonian system 289
2.4.The Riccati equation and feedback optimal control 293
3.Formulation of Stochastic LQ Problems 300
3.1.Statement of the problems 300
3.2.Examples 301
4.Finiteness and Solvability 304
5.A Necessary Condition and a Hamiltonian System 308
6.Stochastic Riccati Equations 313
7.Global Solvability of Stochastic Riccati Equations 319
7.1.Existence:The standard case 320
7.2.Existence:The case C=0,S=0,and Q,G≥0 324
7.3.Existence:The one-dimensional case 329
8.A Mean-variance Portfolio Selection Problem 335
9.Historical Rcmarks 342
Chapter 7.Backward Stochastic Differential Equations 345
1.Introduction 345
2.Linear Backward Stochastic Diffrential Equations 347
3.Nonlinear Backward Stochastic Differential Equations 354
3.1.BSDEs in finite deterministic durations:Method of contraction mapping 354
3.2.BSDEs in random durations:Method of continuation 360
4.Feynman-Kac-Type Formulae 372
4.1.Representation via SDEs 372
4.2.Representation via BSDEs 377
5.Forward-Backward Stochastic Differential Equations 381
5.1.General formulation and nonsolvability 382
5.2.The four-step scheme,a heuristie dcrivation 383
5.3.Several solvablc classes of FBSDEs 387
6.Option Pricing Problems 392
6.1.European call options and the Black-Scholes formula 392
6.2.Other options 396
7.Historical Remarks 398
References 401
Index 433
- 《高含硫气藏开发腐蚀控制技术与实践》唐永帆,张强 2018
- 《钢铁行业污染特征与全过程控制技术研究》周长波等 2019
- 《生活垃圾焚烧飞灰中典型污染物控制技术》朱芬芬等编著 2019
- 《钢铁烧结烟气多污染物过程控制原理与新技术》甘敏,范晓慧著 2019
- 《真菌毒素控制集成解决方案 第二届MycoKey国际真菌毒素大会论文集》冯洁 2018
- 《医疗机构内新型冠状病毒感染预防与控制相关流程》河南省卫生健康委员会编写 2020
- 《工业过程控制及安全技术》王华忠编著 2019
- 《舰艇武器控制中的随机过程应用基础》卢发兴 2019
- 《有源配电网无功补偿与电压控制》(中国)林志超,张勇军,刘轩 2019
- 《蔬菜重金属控制原理与方法》徐卫红著 2019
- 《上班族出走 90年代生涯新主张 事业与休息的平衡观》廖和敏著 1996
- 《阿拉伯-伊斯兰文化史 第3册》埃及艾哈迈德·爱敏著 2019
- 《犯罪学》许桂敏著 2017
- 《会议学与会议管理 第3版》向国敏著 2019
- 《英汉对比在英语翻译教学中的运用研究》郭敏著 2018
- 《阿拉伯-伊斯兰文化史 第7册》埃及艾哈迈德·爱敏著 2019
- 《阿拉伯-伊斯兰文化史 第4册》埃及艾哈迈德·爱敏著 2019
- 《海兰珠传奇》罗敏著 2019
- 《游走于暗夜与光明之间》胡学敏著 2020
- 《李煜》陈敏著 2019
- 《TED说话的力量 世界优秀演讲者的口才秘诀》(坦桑)阿卡什·P.卡里亚著 2019
- 《小手画出大世界 恐龙世界》登亚编绘 2008
- 《近代世界史文献丛编 19》王强主编 2017
- 《课堂上听不到的历史传奇 世界政治军事名人 初中版》顾跃忠等编著 2015
- 《指向核心素养 北京十一学校名师教学设计 英语 七年级 上 配人教版》周志英总主编 2019
- 《365奇趣英语乐园 世界民间故事》爱思得图书国际企业 2018
- 《近代世界史文献丛编 36》王强主编 2017
- 《北京生态环境保护》《北京环境保护丛书》编委会编著 2018
- 《近代世界史文献丛编 11》王强主编 2017
- 《近代世界史文献丛编 18》王强主编 2017