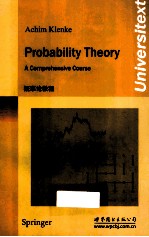
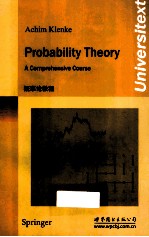
概率论教程PDF电子书下载
- 电子书积分:18 积分如何计算积分?
- 作 者:(德)凯兰克著
- 出 版 社:北京:世界图书北京出版公司
- 出版年份:2012
- ISBN:9787510044113
- 页数:621 页
1 Basic Measure Theory 1
1.1 Classes of Sets 1
1.2 Set Functions 12
1.3 The Measure Extension Theorem 18
1.4 Measurable Maps 34
1.5 Random Variables 43
2 Independence 49
2.1 Independence ofEvents 49
2.2 Independent Random Variables 56
2.3 Kolmogorov's 0-1 Law 63
2.4 Example:Percolation 66
3 Generating Functions 77
3.1 Definition and Examples 77
3.2 Poisson Approximation 80
3.3 Branching Processes 82
4 The Integral 85
4.1 Construction and Simple Properties 85
4.2 Monotone Convergence and Fatou's Lemma 93
4.3 Lebesgue Integral versus Riemann Integral 95
5 Moments and Laws ofLarge Numbers 101
5.1 Moments 101
5.2 Weak Law of Large Numbers 108
5.3 Strong Law of Large Numbers 111
5.4 Speed of Convergence in the Strong LLN 119
5.5 The Poisson Process 123
6 Convergence Theorems 129
6.1 Almost Sure and Measure Convergence 129
6.2 Uniform Integrability 134
6.3 Exchanging Integral and Differentiation 140
7 Lp-Spaces and the Radon-Nikodym Theorem 143
7.1 Definitions 143
7.2 Inequalities and the Fischer-Riesz Theorem 145
7.3 Hilbert Spaces 151
7.4 Lebesgue's Decomposition Theorem 154
7.5 Supplement:Signed Measures 158
7.6 Supplement:Dual Spaces 165
8 Conditional Expectations 169
8.1 Elementary Conditional Probabilities 169
8.2 Conditional Expectations 173
8.3 Regular Conditional Distribution 179
9 Martingales 189
9.1 Processes,Filtrations,Stopping Times 189
9.2 Martingales 194
9.3 Discrete Stochastic Integral 198
9.4 Discrete Martingale Representation Theorem and the CRR Model 200
10 Optional Sampling Theorems 205
10.1 Doob Decomposition and Square Variation 205
10.2 Optional Sampling and Optional Stopping 209
10.3 Uniform Integrability and Optional Sampling 214
11 Martingale Convergence Theorems and Their Applications 217
11.1 Doob's Inequality 217
11.2 Martingale Convergence Theorems 219
11.3 Example:Branching Process 228
12 Backwards Martingales and Exchangeability 231
12.1 Exchangeable Families of Random Variables 231
12.2 Backwards Martingales 236
12.3 De Finetti's Theorem 239
13 Convergence of Measures 245
13.1 A Topology Primer 245
13.2 Weak and Vague Convergence 251
13.3 Prohorov's Theorem 259
13.4 Application:A Fresh Look at de Finetti's Theorem 268
14 Probability Measures on Product Spaces 271
14.1 Product Spaces 272
14.2 Finite Products and Transition Kernels 275
14.3 Kolmogorov's Extension Theorem 283
14.4 Markov Semigroups 288
15 Characteristic Functions and the Central Limit Theorem 293
15.1 Separating Classes of Functions 293
15.2 Characteristic Functions:Examples 300
15.3 Lévy's Continuity Theorem 307
15.4 Characteristic Functions and Moments 312
15.5 The Central Limit Theorem 317
15.6 Multidimensional Central Limit Theorem 324
16 Infinitely Divisible Distributions 327
16.1 Lévy-Khinchin Formula 327
16.2 Stable Distributions 339
17 Markov Chains 345
17.1 Definitions and Construction 345
17.2 Discrete Markov Chains:Examples 352
17.3 Discrcte Markov Processes in Continuous Time 356
17.4 Discrete Markov Chains:Recurrence and Transience 361
17.5 Application:Recurrence and Transience of Random Walks 365
17.6 Invariant Distributions 372
18 Convergence of Markov Chains 379
18.1 Periodicity of Markov Chains 379
18.2 Coupling and Convergence Theorem 383
18.3 Markov Chain Monte Carlo Method 390
18.4 Speed of Convergence 398
19 Markov Chains and Electrical Networks 403
19.1 Harmonic Functions 404
19.2 Reversible Markov Chains 407
19.3 Finite Electrical Networks 408
19.4 Recurrence and Transience 414
19.5 Network Reduction 421
19.6 Random Walk in a Random Environment 427
20 Ergodic Theory 431
20.1 Definitions 431
20.2 Ergodic Theorems 435
20.3 Examples 437
20.4 Application:Recurrence of Random Walks 439
20.5 Mixing 442
21 Brownian Motion 447
21.1 Continuous Versions 447
21.2 Construction and Path Properties 454
21.3 Strong Markov Property 459
21.4 Supplement:Feller Processes 462
21.5 Construction via L2-Approximation 465
21.6 The Space C([0,∞)) 469
21.7 Convergence of Probability Measures on C([0,∞)) 471
21.8 Donsker's Theorem 474
21.9 Pathwise Convergence of Branching Processes 477
21.10 Square Variation and Local Martingales 483
22 Law of the Iterated Logarithm 495
22.1 Iterated Logarithm for the Brownian Motion 495
22.2 Skorohod's Embedding Theorem 498
22.3 Hartman-Wintner Theorem 503
23 Large Deviations 505
23.1 Cramér's Theorem 506
23.2 Large Deviations Principle 510
23.3 Sanov's Theorem 514
23.4 Varadhan's Lemma and Free Energy 519
24 The Poisson Point Process 525
24.1 Random Measures 525
24.2 Properties of the Poisson Point Process 529
24.3 The Poisson-Dirichlet Distribution 535
25 The It? Integral 543
25.1 It? Integral with Respect to Brownian Motion 543
25.2 It? Integral with Respect to Diffusions 551
25.3 The It? Formula 554
25.4 Dirichiet Problem and Brownian Motion 562
25.5 Recurrence and Transience of Brownian Motion 564
26 Stochastic Differential Equations 567
26.1 Strong Solutions 567
26.2 Weak Solutions and the Martingale Problem 576
26.3 Weak Uniqueness via Duality 583
References 591
Notation Index 599
Name Index 603
Subject Index 607
- 《高级英语阅读与听说教程》刘秀梅编著 2019
- 《看图自学吉他弹唱教程》陈飞编著 2019
- 《激光加工实训技能指导理实一体化教程 下》王秀军,徐永红主编;刘波,刘克生副主编 2017
- 《AutoCAD 2019 循序渐进教程》雷焕平,吴昌松,陈兴奎主编 2019
- 《少儿电子琴入门教程 双色图解版》灌木文化 2019
- 《Photoshop CC 2018基础教程》温培利,付华编著 2019
- 《剑桥国际英语写作教程 段落写作》(美)吉尔·辛格尔顿(Jill Shingleton)编著 2019
- 《英语自学进阶教程全6册 3》爱尔兰迪尔德丽出版社著 2019
- 《雅马哈管乐队训练教程 降E调单簧管 分谱》Japan Band Clinic委员会 2019
- 《线性代数简明教程》刘国庆,赵剑,石玮编著 2019
- 《书情书》(德)布克哈德·施皮南(Burkhard Spinnen)著;(德)琳娜·霍文 2019
- 《美国的伤痕 独立战争与美国政治的暴力基因》(德)霍尔格·霍克(Holger Hoock)著 2019
- 《文明的衰落与复兴》张娜责编;陈维政总主编;孙林译者;(德)阿尔伯特·史怀哲 2019
- 《厄特克尔家族》(德)鲁迪格·杨布鲁特著 2018
- 《弗里德里希·李斯特传》朱希滨责编;贾根良,梅俊杰总主编;梅俊杰译者;(德)欧根·文得乐 2019
- 《弗利克家族》(德)托马斯·拉姆什著 2018
- 《剑桥国际英语写作教程 从句子到段落》(美)劳里·布拉斯(Laurie Blass),(美)德德拉·戈登(Deborah Gordon)编著 2019
- 《量子系统的非平衡多体理论》(意)G.斯蒂芬尼茨,(德)R.冯·莱文 2019
- 《香水》李清华译;(德)帕·聚斯金德 2018
- 《无师自通玩转乐器系列 无师自通玩转长笛》胡越菲译;(德)阿尔内·施瓦茨霍兹 2019
- 《TED说话的力量 世界优秀演讲者的口才秘诀》(坦桑)阿卡什·P.卡里亚著 2019
- 《小手画出大世界 恐龙世界》登亚编绘 2008
- 《近代世界史文献丛编 19》王强主编 2017
- 《课堂上听不到的历史传奇 世界政治军事名人 初中版》顾跃忠等编著 2015
- 《指向核心素养 北京十一学校名师教学设计 英语 七年级 上 配人教版》周志英总主编 2019
- 《365奇趣英语乐园 世界民间故事》爱思得图书国际企业 2018
- 《近代世界史文献丛编 36》王强主编 2017
- 《北京生态环境保护》《北京环境保护丛书》编委会编著 2018
- 《近代世界史文献丛编 11》王强主编 2017
- 《近代世界史文献丛编 18》王强主编 2017