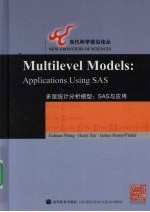
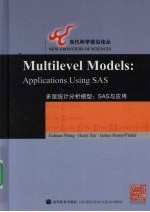
多层统计分析模型 SAS与应用PDF电子书下载
- 电子书积分:11 积分如何计算积分?
- 作 者:王济川,谢海义,(美)James Henry Fisher
- 出 版 社:北京:高等教育出版社
- 出版年份:2009
- ISBN:9787040275681
- 页数:265 页
Chapter 1 Introduction 1
1.1 Conceptual framework of multilevel modeling 1
1.2 Hierarchically structured data 3
1.3 Variables in multilevel data 4
1.4 Analytical problems with multilevel data 6
1.5 Advantages and limitations of multilevel modeling 9
1.6 Computer software for multilevel modeling 11
Chapter 2 Basics of Linear Multilevel Models 13
2.1 Intraclass correlation coefficient(ICC) 13
2.2 Formulation of two-level multilevel models 15
2.3 Model assumptions 17
2.4 Fixed and random regression coefficients 18
2.5 Cross-level interactions 20
2.6 Measurement centering 21
2.7 Model estimation 23
2.8 Model fit,hypothesis testing,and model comparisons 27
2.8.1 Model fit 27
2.8.2 Hypothesis testing 28
2.8.3 Model comparisons 30
2.9 Explained level-1 and level-2 variances 30
2.10 Steps for building multilevel models 33
2.11 Higher-level multilevel models 37
Chapter 3 Application of Two-level Linear Multilevel Models 39
3.1 Data 39
3.2 Empty model 42
3.3 Predicting between-group variation 48
3.4 Predicting within-group variation 53
3.5 Testing random level-1 slopes 57
3.6 Across-level interactions 62
3.7 Other issues in model development 66
Chapter 4 Application of Multilevel Modeling to Longitudinal Data 73
4.1 Features of longitudinal data 73
4.2 Limitations of traditional approaches for modeling longitudinal data 74
4.3 Advantages of multilevel modeling for longitudinal data 75
4.4 Formulation of growth models 75
4.5 Data description and manipulation 77
4.6 Linear growth models 79
4.6.1 The shape of average outcome change over time 80
4.6.2 Random intercept growth models 80
4.6.3 Random intercept and slope growth models 84
4.6.4 Intercept and slope as outcomes 86
4.6.5 Controlling for individual background variables in models 88
4.6.6 Coding time score 89
4.6.7 Residual variance/covariance structures 91
4.6.8 Time-varying covariates 95
4.7 Curvilinear growth models 98
4.7.1 Polynomial growth model 98
4.7.2 Dealing with collinearity in higher order polynomial growth model 100
4.7.3 Piecewise(linear spline)growth model 106
Chapter 5 Multilevel Models for Discrete Outcome Measures 113
5.1 Introduction to generalized linear mixed models 113
5.1.1 Generalized linear models 113
5.1.2 Generalized linear mixed models 115
5.2 SAS Procedures for multilevel modeling with discrete outcomes 116
5.3 Multilevel models for binary outcomes 117
5.3.1 Logistic regression models 117
5.3.2 Probit models 118
5.3.3 Unobserved latent variables and observed binary outcome measures 119
5.3.4 Multilevel logistic regression models 119
5.3.5 Application of multilevel logistic regression models 120
5.3.6 Application of multilevel logit models to longitudinal data 136
5.4 Multilevel models for ordinal outcomes 139
5.4.1 Cumulative logit models 139
5.4.2 Multilevel cumulative logit models 141
5.5 Multilevel models for nominal outcomes 146
5.5.1 Multinomial logit models 146
5.5.2 Multilevel multinomial logit models 147
5.5.3 Application of multilevel multinomial logit models 148
5.6 Multilevel models for count outcomes 154
5.6.1 Poisson regression models 155
5.6.2 Poisson regression with over-dispersion and a negative binomial model 157
5.6.3 Multilevel Poisson and negative binomial models 158
5.6.4 Application of multilevel Poisson and negative binomial models 158
Chapter 6 Other Applications of Multilevel Modeling and Related Issues 175
6.1 Multilevel zero-inflated models for count data with extra zeros 175
6.1.1 Fixed-effect ZIP model 176
6.1.2 Random effect zero-inflated Poisson (RE-ZIP)models 177
6.1.3 Random effect zero-inflated negative binomial(RE-ZINB)models 178
6.1.4 Application ofRE-ZIP and RE-ZINB models 178
6.2 Mixed-effect mixed-distribution models for semi-continuous outcomes 188
6.2.1 Mixed-effects mixed distribution model 189
6.2.2 Application of the Mixed-Effect mixed distribution model 190
6.3 Bootstrap multilevel modeling 195
6.3.1 Nonparametric residual bootstrap multilevel modeling 196
6.3.2 Parametric residual bootstrap multilevel modeling 197
6.3.3 Application of nonparametric residual bootstrap multilevel modeling 198
6.4 Group-based models for longitudinal data analysis 210
6.4.1 Introduction to group-based model 212
6.4.2 Group-based logit model 214
6.4.3 Group-based zero-inflated Poisson(ZIP)model 222
6.4.4 Group-based censored normal models 230
6.5 Missing values issue 237
6.5.1 Missing data mechanisms and their implications 238
6.5.2 Handling missing data in longitudinal data analyses 240
6.6 Statistical power and sample size for multilevel modeling 241
6.6.1 Sample size estimation for two-level designs 242
6.6.2 Sample size estimation for longitudinal data analysis 242
Reference 247
Index 259
- 《水面舰艇编队作战运筹分析》谭安胜著 2009
- 《建筑施工企业统计》杨淑芝主编 2008
- 《分析化学》陈怀侠主编 2019
- 《影响葡萄和葡萄酒中酚类特征的因素分析》朱磊 2019
- 《仪器分析技术 第2版》曹国庆 2018
- 《全国普通高等中医药院校药学类专业十三五规划教材 第二轮规划教材 分析化学实验 第2版》池玉梅 2018
- 《Power BI数据清洗与可视化交互式分析》陈剑 2020
- 《行测资料分析》李永新主编 2019
- 《药物分析》贡济宇主编 2017
- 《土壤环境监测前沿分析测试方法研究》中国环境监测总站编著 2018
- 《SQL与关系数据库理论》(美)戴特(C.J.Date) 2019
- 《魔法销售台词》(美)埃尔默·惠勒著 2019
- 《看漫画学钢琴 技巧 3》高宁译;(日)川崎美雪 2019
- 《思维导图 超好用英语单词书》(中国)王若琳 2019
- 《优势谈判 15周年经典版》(美)罗杰·道森 2018
- 《社会学与人类生活 社会问题解析 第11版》(美)James M. Henslin(詹姆斯·M. 汉斯林) 2019
- 《海明威书信集:1917-1961 下》(美)海明威(Ernest Hemingway)著;潘小松译 2019
- 《学前儿童发展心理学 第2版》刘万伦主编;王文秀,甘卫群,刘万伦编写人员 2019
- 《迁徙 默温自选诗集 上》(美)W.S.默温著;伽禾译 2020
- 《数字影视特效制作技法解析》王文瑞著 2019
- 《全国高等中医药行业“十三五”创新教材 中医药学概论》翟华强 2019
- 《培智学校义务教育实验教科书教师教学用书 生活适应 二年级 上》人民教育出版社,课程教材研究所,特殊教育课程教材研究中心编著 2019
- 《指向核心素养 北京十一学校名师教学设计 英语 七年级 上 配人教版》周志英总主编 2019
- 《习近平总书记教育重要论述讲义》本书编写组 2020
- 《办好人民满意的教育 全国教育满意度调查报告》(中国)中国教育科学研究院 2019
- 《高等数学试题与详解》西安电子科技大学高等数学教学团队 2019
- 《北京生态环境保护》《北京环境保护丛书》编委会编著 2018
- 《教育学考研应试宝典》徐影主编 2019
- 《语文教育教学实践探索》陈德收 2018
- 《家庭音乐素养教育》刘畅 2018