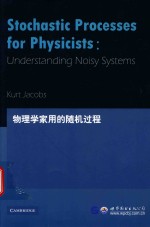
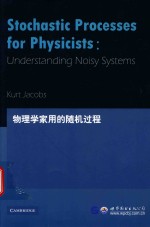
物理学家用的随机过程PDF电子书下载
- 电子书积分:9 积分如何计算积分?
- 作 者:(美)K.雅各布斯著
- 出 版 社:北京/西安:世界图书出版公司
- 出版年份:2018
- ISBN:9787519244668
- 页数:188 页
1 A review of probability theory 1
1.1 Random variables and mutually exclusive events 1
1.2 Independence 4
1.3 Dependent random variables 5
1.4 Correlations and correlation coefficients 6
1.5 Adding independent random variables together 7
1.6 Transformations ofa random variable 8
1.7 The distribution function 10
1.8 The characteristic function 10
1.9 Moments and cumulants 12
1.10 The multivariate Gaussian 13
2 Differential equations 16
2.1 Introduction 16
2.2 Vector differential equations 17
2.3 Writing differential equations using differentials 18
2.4 Two methods for solving differential equations 18
2.4.1 A linear differential equation with driving 20
2.5 Solving vector linear differential equations 21
2.6 Diagonalizing a matrix 23
3 Stochastic equations with Gaussian noise 26
3.1 Introduction 26
3.2 Gaussian increments and the continuum limit 28
3.3 Interlude:why Gaussian noise? 31
3.4 Ito calculus 32
3.5 Ito’s formula:changing variables in an SDE 35
3.6 Solving some stochastic equations 37
3.6.1 The Ornstein-Uhlenbeck process 37
3.6.2 The full linear stochastic equation 39
3.6.3 Ito stochastic integrals 40
3.7 Deriving equations for the means and variances 41
3.8 Multiple variables and multiple noise sources 42
3.8.1 Stochastic equations with multiple noise sources 42
3.8.2 Ito’s formula for multiple variables 44
3.8.3 Multiple Ito stochastic integrals 45
3.8.4 The multivariate linear equation with additive noise 48
3.8.5 The full multivariate linear stochastic equation 48
3.9 Non-anticipating functions 51
4 Further properties of stochastic processes 55
4.1 Samplepaths 55
4.2 The reflection principle and the first-passage time 57
4.3 The stationary auto-correlation function,g(τ) 59
4.4 Conditional probability densities 60
4.5 The power spectrum 61
4.5.1 Signals with finite energy 63
4.5.2 Signals with finite power 65
4.6 White noise 66
5 Some applications of Gaussian noise 71
5.1 Physics:Brownian motion 71
5.2 Finance:option pricing 74
5.2.1 Some preliminary concepts 75
5.2.2 Deriving the Black-Scholes equation 78
5.2.3 Creating a portfolio that is equivalent to an option 81
5.2.4 The price of a“European”option 82
5.3 Modeling multiplicative noise in real systems:Stratonovich integrals 85
6 Numerical methods for Gaussian noise 91
6.1 Euler’s method 91
6.1.1 Generating Gaussian random variables 92
6.2 Checking the accuracy of a solution 92
6.3 The accuracy of a numerical method 94
6.4 Milstein’s method 95
6.4.1 Vector equations with scalar noise 95
6.4.2 Vector equations with commutative noise 96
6.4.3 General vector equations 97
6.5 Runge-Kutta-like methods 98
6.6 Implicit methods 99
6.7 Weak solutions 99
6.7.1 Second-order weak methods 100
7 Fokker-Planck equations and reaction-diffusion systems 102
7.1 Deriving the Fokker-Planck equation 102
7.2 The probability current 104
7.3 Absorbing and reflecting boundaries 105
7.4 Stationary solutions for one dimension 106
7.5 Physics:thermalization of a single particle 107
7.6 Time-dependent solutions 109
7.6.1 Green’s functions 110
7.7 Calculating first-passage times 111
7.7.1 The time to exit an interval 111
7.7.2 The time to exit through one end of an interval 113
7.8 Chemistry:reaction-diffusion equations 116
7.9 Chemistry:pattern formation in reaction-diffusion systems 119
8 Jumpprocesses 127
8.1 The Poisson process 127
8.2 Stochastic equations for jump processes 130
8.3 The master equation 131
8.4 Moments and the generating function 133
8.5 Another simple jump process:“telegraph noise” 134
8.6 Solving the master equation:a more complex example 136
8.7 The general form of the master equation 139
8.8 Biology:predator-prey systems 140
8.9 Biology:neurons and stochastic resonance 144
9 Levy processes 151
9.1 Introduction 151
9.2 The stable Levy processes 152
9.2.1 Stochastic equations with the stable processes 156
9.2.2 Numerical simulation 157
9.3 Characterizing all the Levy processes 159
9.4 Stochastic calculus for Levy processes 162
9.4.1 The linear stochastic equation with a Levy process 163
10 Modern probability theory 166
10.1 Introduction 166
10.2 The set of all samples 167
10.3 The collection of all events 167
10.4 The collection of events forms a sigma-algebra 167
10.5 The probability measure 169
10.6 Collecting the concepts:random variables 171
10.7 Stochastic processes:filtrations and adapted processes 174
10.7.1 Martingales 175
10.8 Translating the modern language 176
Appendix A Calculating Gaussian integrals 181
References 184
Index 186
- 《催化剂制备过程技术》韩勇责任编辑;(中国)张继光 2019
- 《新编高中物理竞赛教程习题全解》钟小平主编;钟小平,倪国富,曹海奇编写 2019
- 《数学物理方法与仿真 第3版》杨华军 2020
- 《绿色过程工程与清洁生产技术 张懿院士论文集精选 上》《绿色过程工程与清洁生产技术》编写组编 2019
- 《化工传递过程导论 第2版》阎建民,刘辉 2020
- 《中学物理奥赛辅导:热学 光学 近代物理学》崔宏滨 2012
- 《长江口物理、化学与生态环境调查图集》于非 2019
- 《大学物理简明教程 下 第2版》施卫主编 2020
- 《慢性呼吸系统疾病物理治疗工作手册》(荷)瑞克·考斯林克(RikGosselink) 2020
- 《医学物理学》洪洋 2020
- 《TED说话的力量 世界优秀演讲者的口才秘诀》(坦桑)阿卡什·P.卡里亚著 2019
- 《小手画出大世界 恐龙世界》登亚编绘 2008
- 《近代世界史文献丛编 19》王强主编 2017
- 《课堂上听不到的历史传奇 世界政治军事名人 初中版》顾跃忠等编著 2015
- 《指向核心素养 北京十一学校名师教学设计 英语 七年级 上 配人教版》周志英总主编 2019
- 《365奇趣英语乐园 世界民间故事》爱思得图书国际企业 2018
- 《近代世界史文献丛编 36》王强主编 2017
- 《北京生态环境保护》《北京环境保护丛书》编委会编著 2018
- 《近代世界史文献丛编 11》王强主编 2017
- 《近代世界史文献丛编 18》王强主编 2017